Estimating & Understanding Architectural Risk
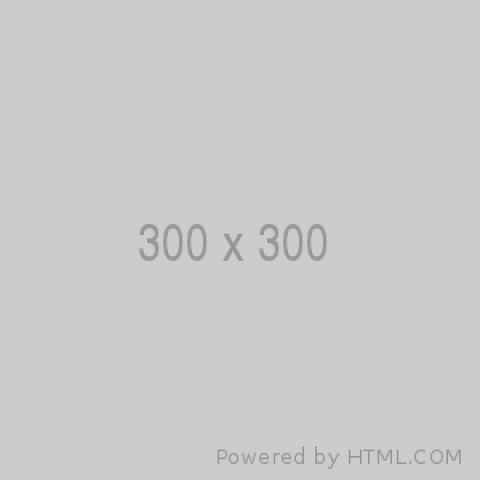
While new technologies present tremendous promise for doing energy efficient computing, designing an efficient computer system in an era of rapidly evolving application behaviors and significant technology shifts involves taking on risk that a design will fail to meet its performance or efficiency goals. While risk assessment and management are expected in both business and investment, computer system designers typically treat such risk as independent to questions of performance and energy efficiency. Instead they tend to construct and evaluate models of the computing systems they are creating using concrete values and most only estimate risk in a back-of-the-envelope way.
UCSB Computer Science graduate student Weilong Cui and Professor Tim Sherwood have been developing a new approach to this problem that promises to help smooth the transition from energy efficiency in the lab to energy efficient in production. Their upcoming paper, to be presented in the in the 2017 International Symposium on Microarchitecture, is the first step in towards a solution. As hardware and software characteristics become uncertain (i.e. samples from a distribution), they demonstrate that the resulting performance distributions quickly grow beyond even an expert's ability to reason about with intuition alone. They show that knowledge of the performance distribution can be used to significantly improve both the average case performance and minimize the risk of under-performance. By developing an automated framework that can be used to quantify the design space where trade-offs between expected performance and the "tail" of performance are most acute they provide new insights supporting computer design under uncertainty. A copy of their manuscript and more information about the project is available here, and on Professor Sherwood's website.